Control, planning and decision-making constitute three fundamental building blocks to execute any given operation in an optimal way with respect to time, cost, resources, and quality. Typically, these building blocks are put in a hierarchical context, with a controller at the bottom layer, a planning solution at the middle layer, and a decision support system at the top layer. These layers exchange information such as measured and desired values with varying sampling rates and amounts of data. We use their hierarchical structure to reduce the complexity of holistic process control through well-defined and well-separated tasks. We strive to push the performance of each layer beyond current state-of-the-art solutions by systematically exploiting maximum knowledge of and information about the underlying processes. For the bottom layer, we develop advanced, real-time-capable control and optimisation algorithms that minimise errors between process-specific variables and their desired values while suppressing disturbances and stabilising the regarded system. Moreover, we supply higher layers, e.g., the planning and decision-making instance, with adequate information provided by the subordinate control instance. For the planning instance, we research new methods to calculate optimal desired values of the subordinate controllers in the form of trajectories and specific control schemes. Additionally, we research and develop highly efficient and optimal solutions for sequence and scheduling problems at the decision-making layer.
Mathematical models which enable accurate prediction of system behaviour constitute the basis for designing robust and adaptable solutions across all hierarchical layers of advanced automation systems. Finally, we employ adaptive and learning concepts to continuously improve model performance.
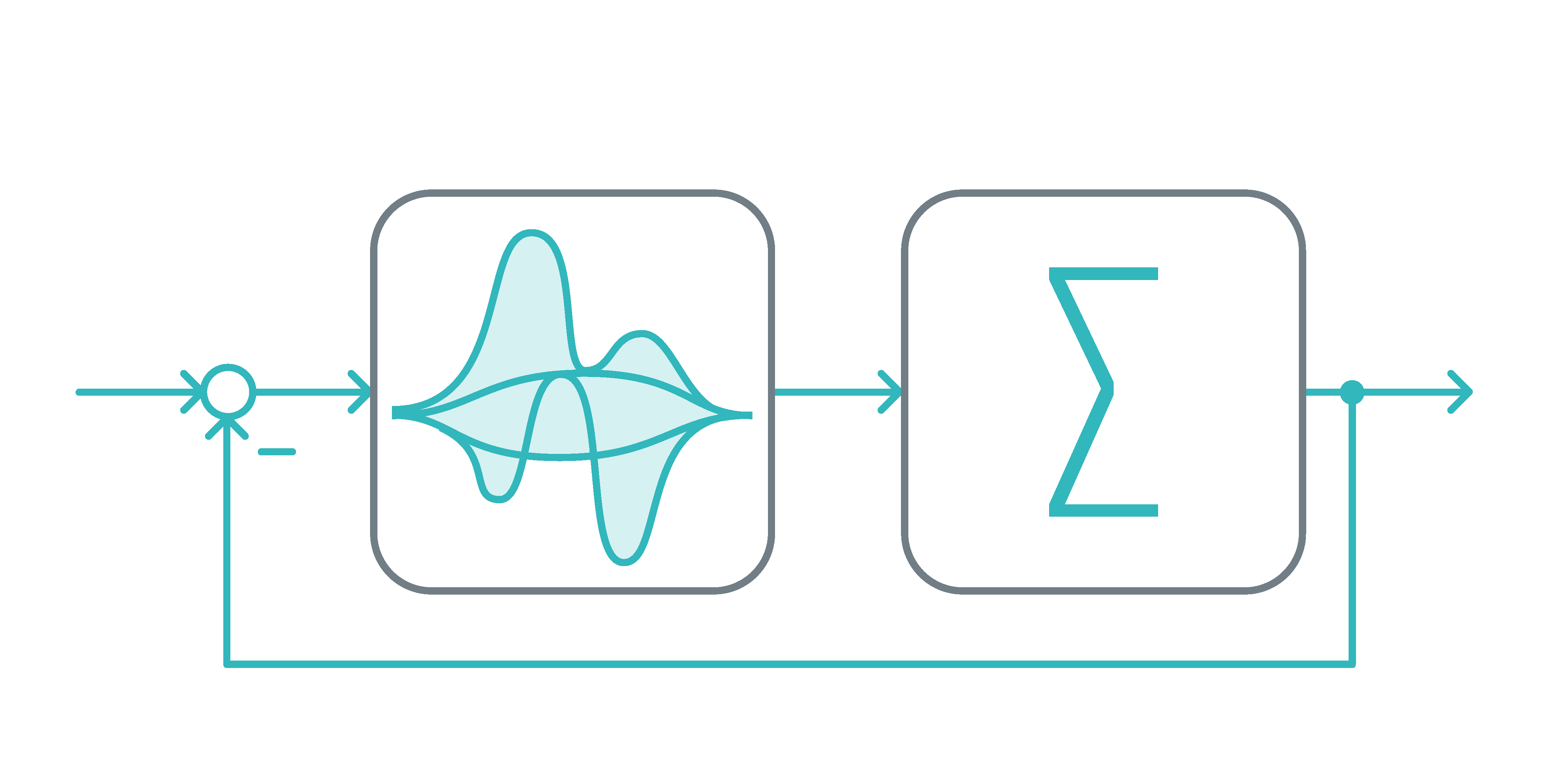
Control & Optimisation
Linear control concepts (e.g., PID) still dominate the current industrial standard for the control of real-world complex dynamical systems. Linear controllers disallow systematic consideration of the system-inherent properties like nonlinearities and couplings. As a result, their attainable performance is fundamentally limited. We overcome this hurdle by developing tailored, model-based control algorithms taking system properties and partner-specific requirements into account.
Among the available solutions are linear and nonlinear feed-forward and feed-back control, adaptive and learning multivariate control as well as model-predictive multivariate control of nonlinear systems with constraints. Leveraging our own solvers, we use model-predictive control even for sophisticated real-time applications. In addition, we achieve significantly improved performance and robust solutions of manufacturing processes or drive systems through problem-specific optimisation of control parameters.
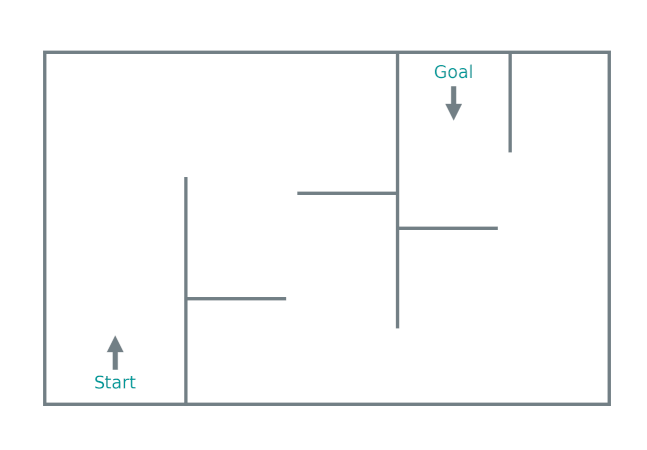
Planning &
Decision-Making
Initially, multiple desired trajectories may be feasible for a given control task. We develop optimisation-based planning and decision-making algorithms to generate and select the most appropriate trajectory considering the system characteristics as well as the physical and process-specific constraints. These algorithms may push systems to their respective reasonable limits. Our research comprises optimisation of static operating points as well as path and trajectory planning for small- and large-scale manipulators, taking into account obstacles to enable safe, autonomous operation. Moreover, we research sequence and schedule optimisation in manufacturing systems to boost product quality, resource efficiency, and throughput.
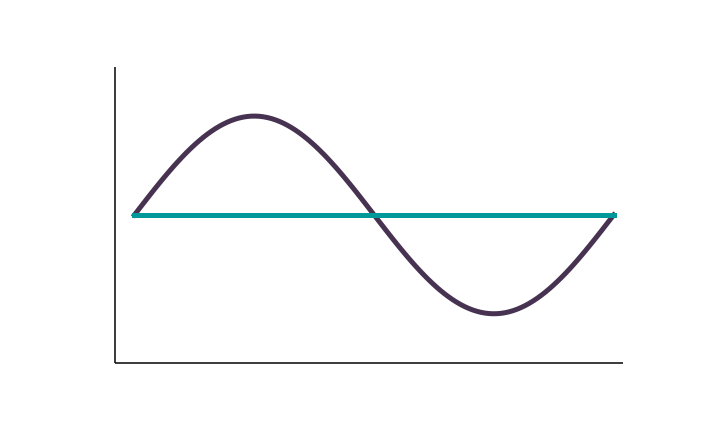
Prediction & Learning
The complexity of automation solutions is directly affected by ever-changing system environments which may generally lead to deterioration of control performance, reproducibility, and process stability, eventually resulting in diminishing revenue and competitiveness. We address this challenge by developing learning strategies based on adaptive, robust control theory and data-driven methods to adapt our model-based control and planning concepts. Furthermore, we combine this approach with our results from system identification and parameter estimation to continuously increase the model accuracy and, thereby, the control performance.
Linked Solutions
Research Groups Involved